How to Achieve Sustainable AI Value: Moving from Proof of Concept to Production
Transitioning AI projects from Proof of Concept (PoC) to production is crucial for unlocking AI's full potential and driving sustainable business value. This requires strategic alignment, robust portfolio management, scalability considerations, and effective risk management, with strong leadership to champion AI initiatives and foster innovation.
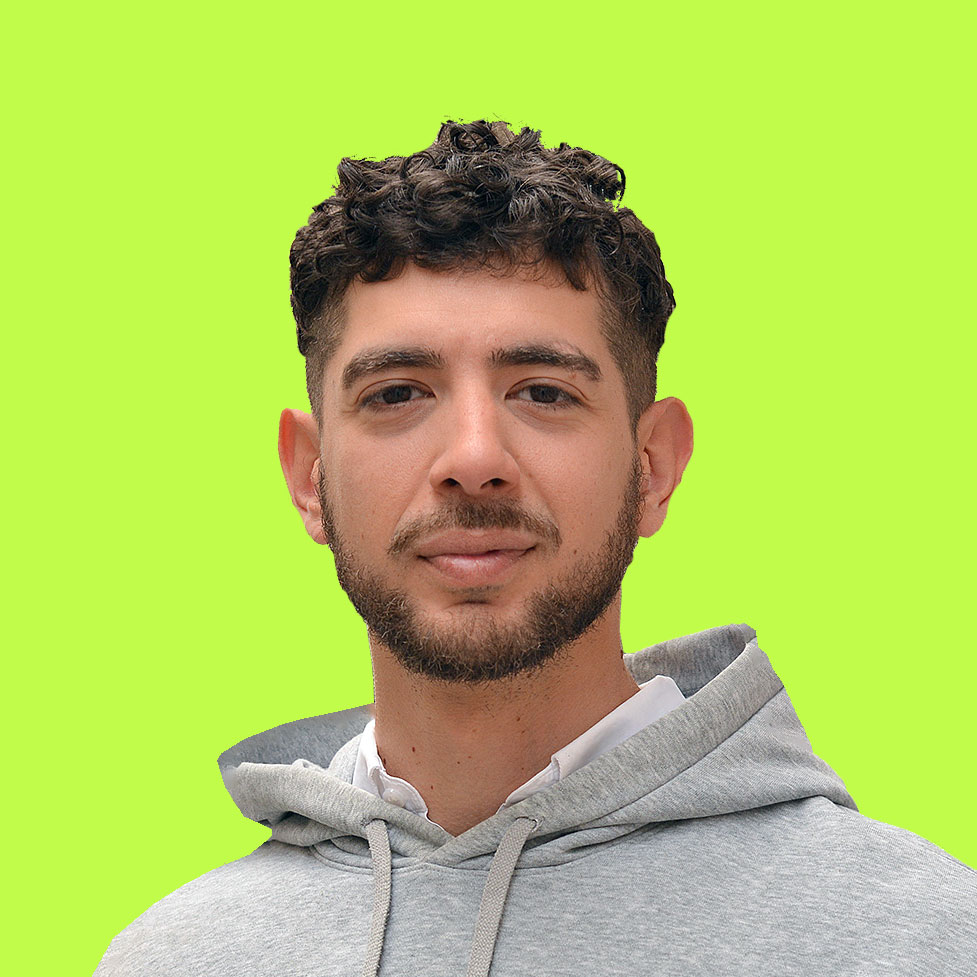
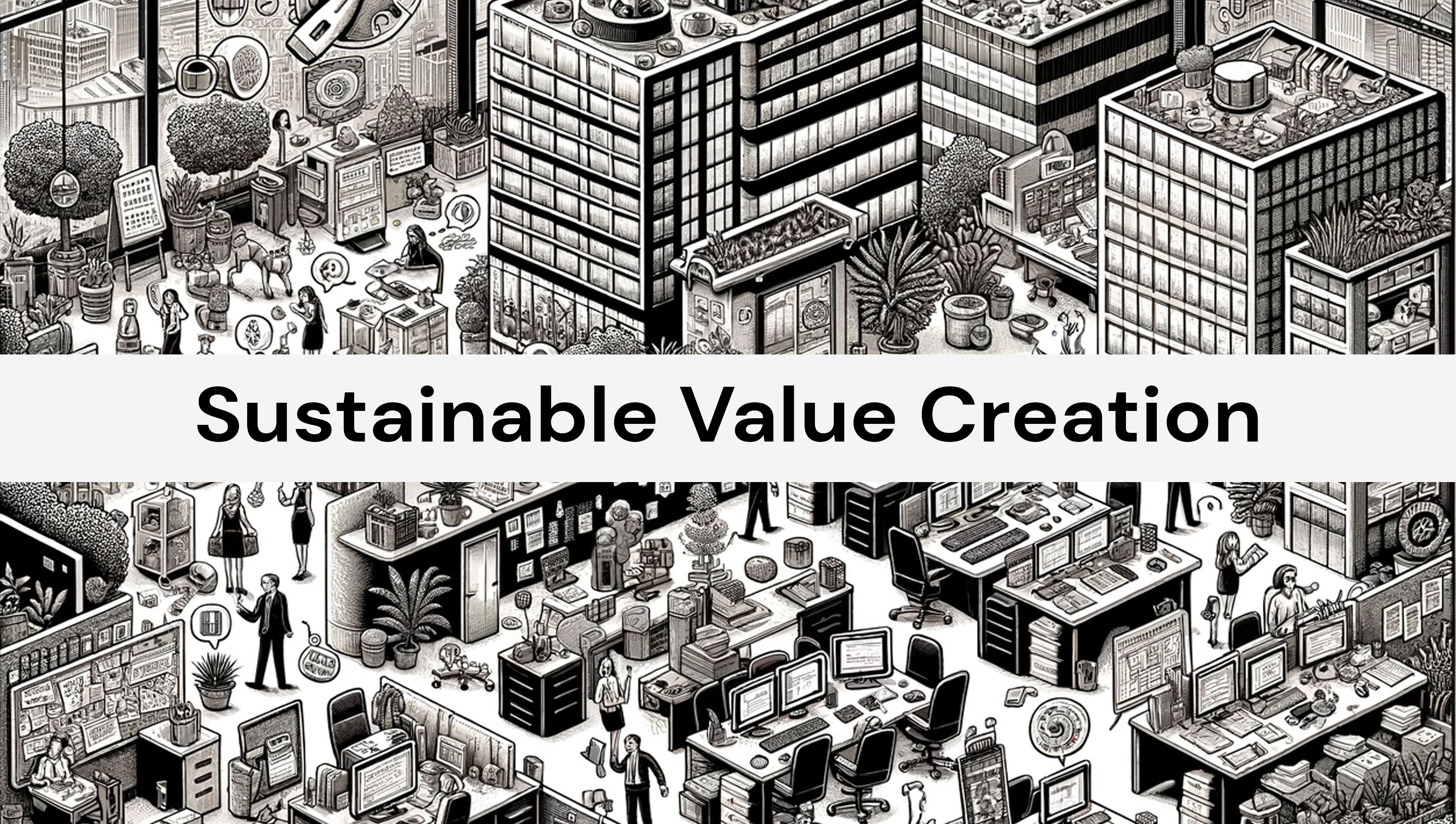
Intro & Summary
Imagine you've just scored tickets to the hottest concert of the year. You arrive at the venue, excitement building, only to be stuck in the lobby, unable to enter the main event. Frustrating, right? That's how many companies feel about their AI initiatives.
AI is more than just another tech trend—it's the VIP pass to the future of business. It has the potential to revolutionize industries, spark innovation, and unlock entirely new business models. Yet, despite this promise, many companies are stranded at the Proof of Concept (PoC) stage, unable to make the leap into full-scale production.
The roadblocks are familiar: lack of strategic alignment, insufficient resources, and the struggle to integrate AI solutions seamlessly into existing operations. It's like having a killer playlist but no sound system to play it on. Overcoming these challenges requires a game plan that aligns AI projects with business strategy, scales efficiently, and manages risks effectively.
Understanding the Practitioner Level
The Practitioner level in AI maturity represents a stage where companies have moved beyond initial experimentation and are actively deploying AI solutions. At this level, the focus shifts to systematic implementation and integration of AI projects into the core business processes.
Characteristics of the Practitioner Level:
- Clear AI vision aligned with corporate objectives.
- Established processes for identifying and prioritizing AI use cases.
- Infrastructure and resources dedicated to scaling AI solutions.
- Continuous monitoring and improvement of AI deployments.
Importance of Systematic Implementation:Systematic implementation is crucial to ensure that AI initiatives are sustainable and scalable. This involves not only the technical aspects of AI but also organizational readiness, governance structures, and a culture that supports continuous learning and adaptation.
Key Steps to Move AI Projects to Production
To successfully transition AI projects from PoC to production, companies must focus on several key areas:
- Strategic Alignment:
- Align AI projects with the overall business strategy to ensure they contribute to the company's long-term goals.
- Define clear objectives and key performance indicators (KPIs) for AI initiatives to measure their impact and success.
- Portfolio Management:
- Develop a portfolio of AI use cases that are prioritized based on their potential value and feasibility.
- Use a systematic approach to assess and select use cases, considering factors such as economic impact, ease of implementation, and strategic relevance.
- Scalability Considerations:
- Design AI solutions that are scalable and can be integrated into existing business processes and systems.
- Ensure robust data infrastructure and pipeline management to support large-scale AI deployments.
- Adopt MLOps (Machine Learning Operations) practices to manage the lifecycle of AI models, including deployment, monitoring, and maintenance.
- Risk Management:
- Address risks related to data governance, cybersecurity, and AI ethics.
- Implement frameworks to ensure transparency and accountability in AI decision-making processes.
- Develop contingency plans to handle potential failures or biases in AI systems.
Case Studies and Examples
Example 1: Siemens AI LabSiemens has successfully transitioned AI projects from PoC to production by fostering a culture of innovation and collaboration. The Siemens AI Lab supports internal teams in developing tangible AI prototypes quickly through use case sprints. This approach has enabled Siemens to integrate AI solutions across various business units, driving significant improvements in process efficiency and customer satisfaction.
Example 2: AntGroup’s 3-1-0 Digital Lending ProductAntGroup’s digital lending product, which allows customers to complete loan applications in 3 minutes and receive approval in 1 second with zero human involvement, exemplifies successful AI implementation at scale. By leveraging AI to automate core operations, AntGroup has dramatically increased its customer base and operational efficiency.
Lessons Learned:
- Successful AI implementation requires a clear strategic vision and strong leadership commitment.
- Cross-functional collaboration and a dedicated team are essential for managing AI projects effectively.
- Continuous learning and adaptation are crucial to keep pace with the evolving AI landscape.
Conclusion
Transitioning AI projects from PoC to production is a critical step for companies aiming to unlock the full potential of AI. By aligning AI initiatives with business strategy, managing a portfolio of AI use cases, ensuring scalability, and addressing risks, companies can overcome common challenges and drive sustainable AI value.
C-level executives play a pivotal role in this process by championing AI initiatives, fostering a culture of innovation, and ensuring that AI projects are strategically aligned with the company's goals. Embracing these practices will enable businesses to harness the transformative power of AI and achieve long-term competitive advantage.
Other Posts
Assess your AI Maturity. Increase your organizations AI Value
Understand your organization's current maturity level & find out how to create more AI Value.