How not to screw up your first steps in your AI journey
A practical guide for business leaders considering AI integration focused on cutting through the hype and avoiding rookie mistakes
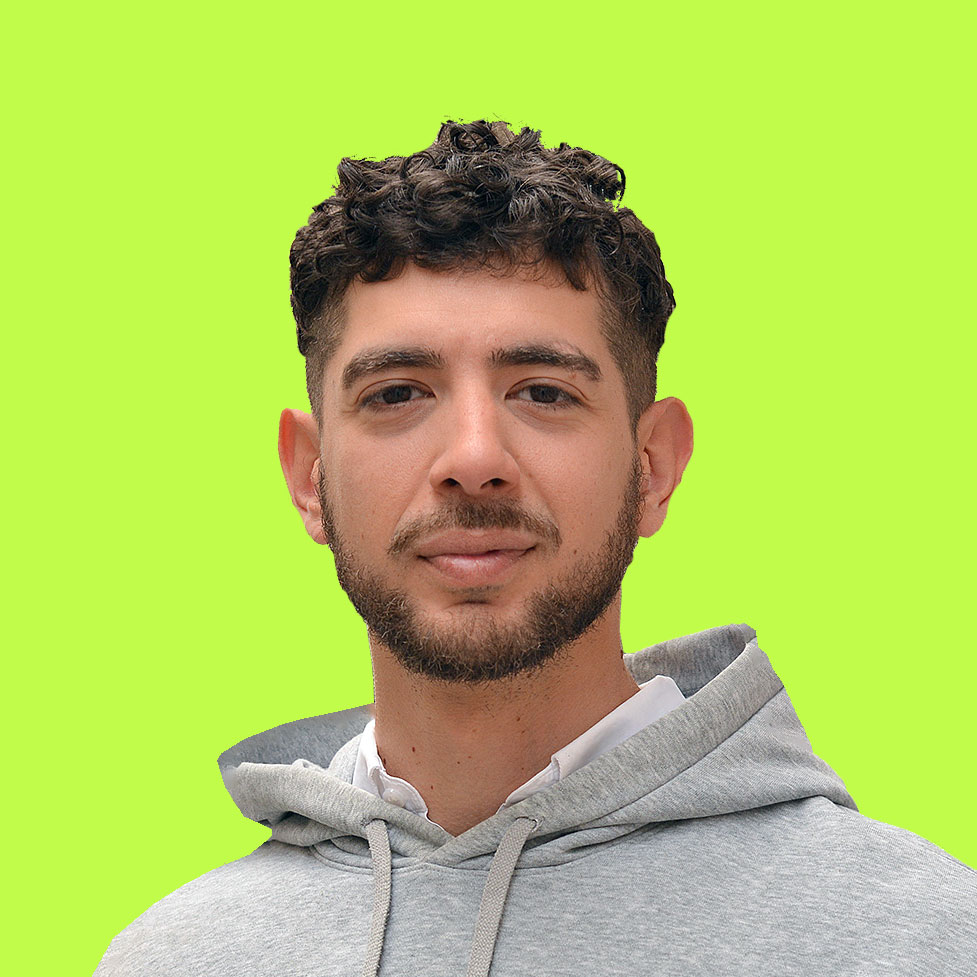
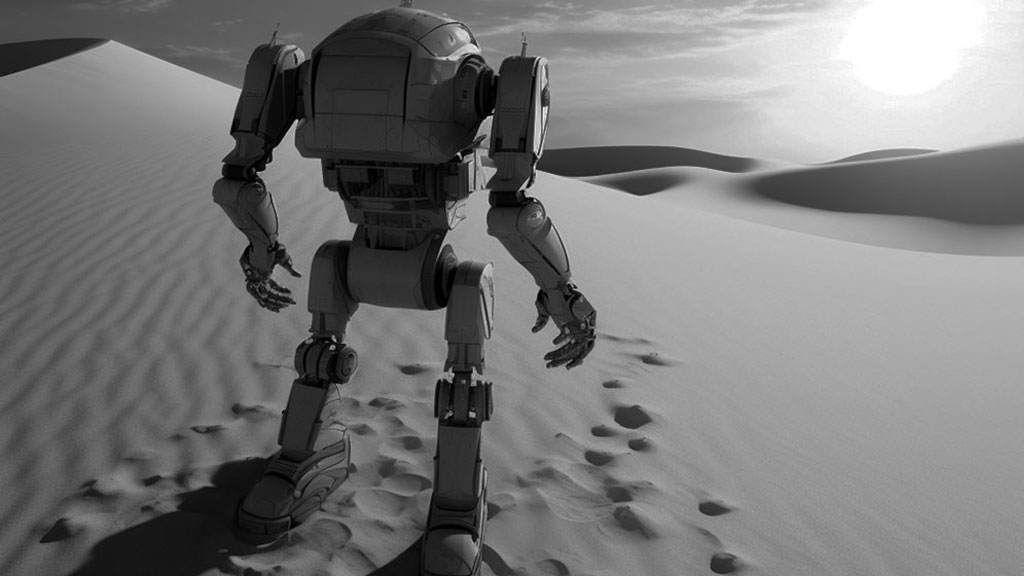
Intro: Embrace AI, don’t fight against it
In the mid to late 1990s, as the internet began to gain traction, many were doubtful of its significance, but now it's an integral part of our lives. Fast forward to the emergence of social media, there was a similar wave of doubt, yet today the global daily average time spent on social media is 2 hours and 31 minutes. As Generative AI begins to make its mark, history seems to be repeating itself. Spoiler alert: We're likely to see Generative AI & its use cases become as commonplace as the internet and social media in due course.
In 2023, AI, especially Generative AI, and LLMs, is reshaping our daily lives, products, and business operations. At Lazy, we continually address questions arising from an overflow of data, misconceptions, and misinformation. Through this article, we aim to guide entrepreneurs, business executives, and leaders who are newcomers to AI, emphasizing the importance of informed knowledge to navigate and thrive. Join us as we dive into the basics, providing key insights. Arming yourself with information is crucial to dodging pitfalls and succeeding in this era.
AI, ML, LLMs: What is the difference?
Think of Artificial Intelligence (AI) as the entire nervous system of an organism. Within this 'nervous system,' Machine Learning (ML) is akin to the brain's ability to form memories and learn from experiences. Large Language Models (LLMs) serve as the specialized language centers within the brain, focused exclusively on understanding and generating text. Just as each part of the nervous system has its role and limitations, so do AI, ML, and LLMs within the broader framework of artificial intelligence.
Much like your brain, these AI systems build a model of the world. However, unlike your brain, they tend to be reasonably brittle and lack the ability to 'learn on the fly.' Instead of experiencing the rich interactions with the world that you might, these systems often interact solely with the world of text.
At its core, an LLM is a statistical model designed to predict what text should come next, given some initial text. Though this sounds straightforward, because these models have been trained on immense amounts of data, they turn out to be quite powerful and flexible.
What can we use LLMs for?
On the highest level, large language models (LLMs) like ChatGPT can be used for problems that require:
- Generating text
- Searching text
- Summarizing text
- Agents that take natural language instructions
How do these translate to business use cases? Here are a few common use cases along with their LLM solutions.
Pre-training & training models: basic concepts
Just as a child goes through various stages of learning, AI models also undergo a formative period called the 'training phase.' In this phase, the AI model is fed a vast amount of data to build its 'knowledge.' There are two steps to this: pre-training, where the model learns the basics, like language structure or pattern recognition, and training, where it gets specialized knowledge tailored to specific tasks. However, once this phase is over, unlike humans, these AI models cease to learn. Their 'educational journey' comes to a standstill, and they apply what they've learned to answer queries, make predictions, or perform tasks.
Can models learn new skills after they are created/released?
Models cannot learn new things. Technically LLMs cannot “learn on the fly”, because the models are a snapshot from the point at which they are trained. However, with the right prompts, the model can generate surprisingly intuitive and useful responses, making it appear to be learning what you want.
Imagine you’re given a machine that you can operate, let’s say it’s a combination of an airplane, car, and boat. It’s 100X more powerful than any machine ever produced on Earth. Instead of the usual airplane cockpit that’s a few meters across, the control panel of this machine is hundreds of meters across.
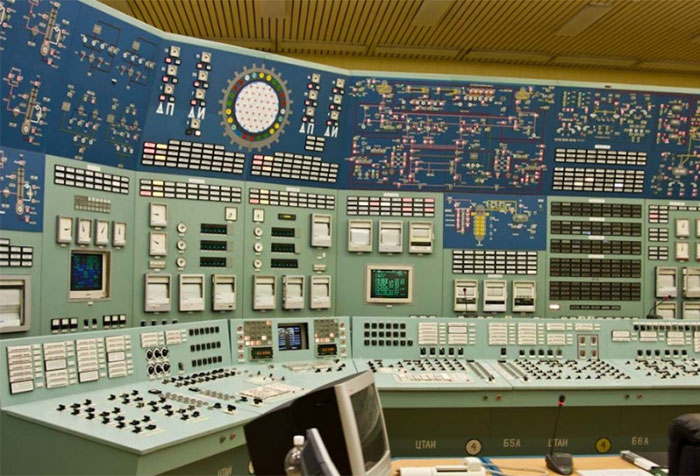
As you learn to operate this machine, you can perform amazing feats with it! But the machine itself never changes, instead, the learning is with your ability to operate it.
Such is the case when using LLMs as you learn to “prompt engineer” them to enable them to perform the tasks you need them to.
What about fine-tuning?
Maybe you heard about fine-tuning. And you may be asking yourself: Doesn’t that teach the model new things? Not exactly. Fine-tuning is more like deriving a brand new model from an existing model, where the new fine-tuned model might be better at some things than the original model, but worse at other things.
Continuing with the super powerful “everything machine” analogy, let’s say you try to use it to drill a huge hole in the bottom of the ocean. However, even after studying the manuals and learning how to control the machine as best as you can, it’s just not that great at drilling.
You go back to the makers of this machine and ask them to rebuild the machine so that it’s good at underwater drilling, even if it will sacrifice its performance on other tasks. After weeks of development and paying the makers a lot of money, they come back to you with your brand-new underwater airplane drilling machine. Voilà!
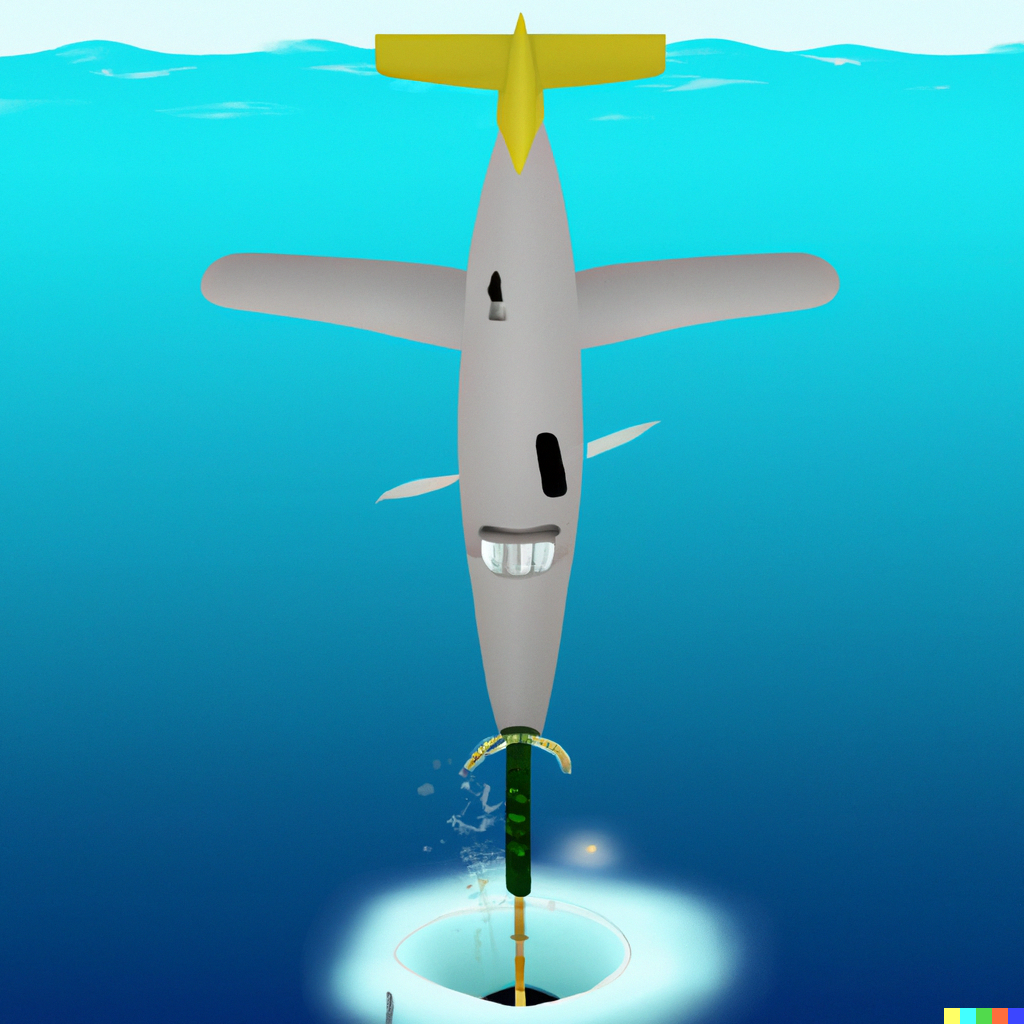
Unfortunately, they had to remove the wheels, so the machine no longer functions as a car.
When to do fine-tuning?
First, try prompt engineering. Try to solve your problem with prompt engineering. There are many different prompt engineering strategies that can help you get the most out of a general model, such as providing specific examples to the model. This is called “Few-shot prompting”. Here are some criteria that may indicate it’s time for you to consider fine-tuning:
- You can’t get the required quality on prompt engineering alone
- Your interactions with the model are in a highly specialized domain with a very specific vocabulary. Law and medicine would be typical examples.
Risks and costs of tuning
Fine-tuning is a powerful technique, but it has costs and risks:
- Collecting high-quality data is time-consuming and expensive.
- Fine-tuning can require specialized technical knowledge.
- Done incorrectly, you might end up with worse results than a general model.
So before embarking on a mission to create a better model via fine-tuning, make sure you actually need it.
T-shirt size pricing:
- Prompt-Engineering: Small (S)
- Fine-tuning: Medium (M)
- Pre-Training: Extra extra large (XXL)
GDPR compliancy
Finally, the most interesting topic in Germany: is GDPR!
Current AI regulations (The “AI Act”) primarily target AI model companies (like OpenAI -ChatGPT), not those implementing the models. However, it's crucial to stay up-to-date, as regulations may become clearer and more specific in the near future.
The GDPR officer of each company needs to be heavily involved in the process of implementing new technologies, and the ultimate responsibility for GDPR compliance falls on them. Nonetheless, here are a few important facts to consider:
- Legal Considerations: Ensure a lawful basis for processing personal data, such as user consent or fulfilling a contract. Conduct a Data Protection Impact Assessment if the feature poses high data regarding privacy risks. Have processes to handle user rights requests, such as data access or erasure.
- Data Management: Only collect and process necessary personal data. Establish policies for data storage and retention, keeping data only as long as necessary. Ensure agreements are in place when using third-party services like GPT-4 API.
- Transparency: Provide clear information to users about their data processing, including updating privacy notices.
- Compliance Monitoring: Conduct regular audits and reviews of data processing activities for ongoing GDPR compliance. Ensure safeguards for data transfers outside the EEA, like standard contractual clauses.
- Security Measures: Implement security measures to protect personal data, including encryption, access controls, and regular data backups.
Data privacy
There is some talk about the risks of sending data to OpenAI, but let's consider the context. It's similar to having your emails on Gmail or Google One. OpenAI is a 27 billion USD company, so they have substantial resources for security. However, if you prefer not to send data to OpenAI, we have the option to self-host the models. And this leads us to our next point.
Self-hosted open source models vs proprietary-hosted models
Choosing between self-hosted open-source models and proprietary hosted models presents a series of trade-offs. Proprietary-hosted models provide ease of use, seamless updates, and streamlined interfaces. They're the "plug-and-play" choice, perfect for businesses without a deep bench of technical expertise. However, they may offer limited customization and potentially tie companies to ongoing subscription costs. In contrast, self-hosted open-source models offer significant control and customization, ideal for businesses with unique needs or those looking to avoid vendor lock-ins. But they come with their challenges: a potentially steep learning curve, the initial setup demands, and the onus of managing updates and maintenance.
T-shirt size pricing:
- Proprietary hosted models: Small (S)
- Self-hosted open-source models: Extra Large (XL)
Extra misconceptions and limitations
Last but not least, here are some other extra misconceptions and limitations we get asked often, so we compiled them here.
Inherent Understanding: Some believe LLMs genuinely understand the content they generate. In reality, they don't "understand" in the way humans do; they identify patterns in data and replicate them.
LLMs Always Give Correct Answers: Some users might assume that LLMs are infallible or always produce correct answers. However, they can provide incorrect or misleading information, especially if their training data had biases or inaccuracies. This is known as “hallucination”. There are techniques available to minimize hallucination if it’s inappropriate for your application. In some cases, where you are trying to generate content, hallucination can actually be a good thing as the LLM can come up with wildly creative ideas!
Unbiased Outputs: Despite their vast training data, LLMs can still produce biased or politically incorrect outputs. They reflect the data they were trained on, which can encompass societal biases. Recent research has shown that different models have different political biases.
Generalization Across All Domains: LLMs, despite their vast knowledge base, may not be experts in super-niche or very recent topics. Their knowledge and accuracy can vary across domains. This is where fine-tuning comes in, more on that below.
Limitless Creativity: While LLMs can generate creative content, their "creativity" is based on the patterns they've seen in the training data. They don't have innate creativity or novel thinking in the same way humans do.
Sometimes GUIs are better: Text is a universal human interface, but it’s not always the most efficient approach. Before jumping into LLMs as a replacement for your GUI, work with a UX designer to make sure it’s the best interface for your particular application.
Key takeaways & closing thoughts
That wraps up this blog post. As we conclude this guide, let's recap the essential concepts and insights we've covered:
- Get to know the basics: If needed dig deeper into what AI is, models, training models, and fine-tuning, before execution.
- Embrace Change: Dive into the potential of AI with an open mind. Explore various use cases, be imaginative, and embrace the transformative possibilities.
- Start, Learn, and Grow: Embrace the KISS principle — "Keep It Simple, Stupid." Adopt the Lean Startup mindset: initiate with minimalistic steps and swiftly adjust. Although the learning curve might be challenging, it's a valuable part of the journey. Take feedback from your users, iterate, and consistently enhance your AI product.
- Personalize Your Approach: Assess your specific needs and choose the right model. From building to fine-tuning, make decisions that align with your current requirements and goals.
- Balance Security, Trust, and Innovation: Protecting privacy and ensuring GDPR compliance are non-negotiable. Build a foundation of trust with your users, while continuing to innovate responsibly.
- Security is Paramount: From IP protection to data security, prioritize safeguarding your business and your users' information.
We hope this blog post has provided you with valuable insights into the world of AI and LLMs. We'd love to hear your thoughts and continue the conversation!
If you have any questions or need any help please reach out to us here.
Thank you for reading
Luciano Radicce & Traun Leyden
Other Posts
Assess your AI Maturity. Increase your organizations AI Value
Understand your organization's current maturity level & find out how to create more AI Value.